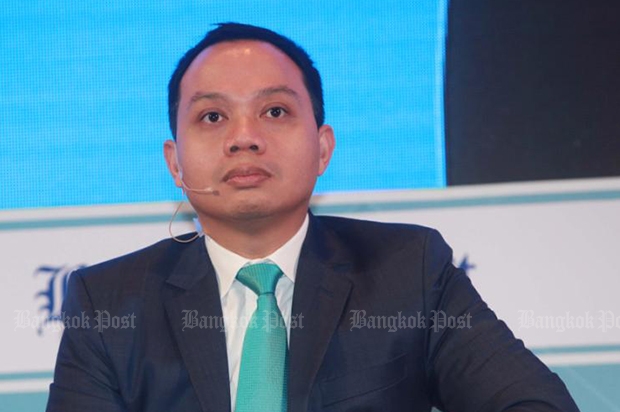
Now that the IT industry has begun to assimilate cloud, mobile, social business and big data technologies, we at IDC Financial Insights anticipate the emergence of even more disruptive technologies.
Among these new technologies we track, cognitive systems stand out as one to watch in the short term. The technology has already been tried and tested by the region's leading banks, and leading vendors have made bold research and development investments. Therefore, sooner rather than later, the use of cognitive systems (some refer to it as machine learning) will become more prevalent in Thai financial services -- accelerating digital transformation in banks, insurance and capital market firms.
Cognitive systems are systems that observe, learn, analyse, offer suggestions and even create new ideas. They are made up of many elements that include machine learning, probabilistic computing, natural language processing, and constant generation and evaluation of hypotheses. The system can understand the context of the situation and make recommendations based on what is the probable and the next best outcome.
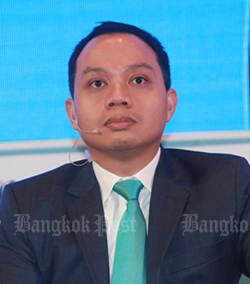
Michael Araneta
Cognitive systems can be important at several levels of the financial institution.
In the back office, it can be beneficial in machine-to-human interaction, optimised processes and services orchestration (especially the IT helpdesk). We believe that IT groups will experience the first wave of benefits as cognitive-based case management systems reduce the manual effort currently required to manage trouble tickets -- although this number might be slightly lower in Thailand. Also, the adoption of cognitive systems will help IT executives streamline operations and create enterprise-wide utilities that improve the IT efficiency of the institution.
It can introduce better decisions in the middle office, which means improved business insights, improved customer insights and better risk/pricing decisions. Cognitive systems will be used in improving risk, product, and pricing models, thus resolving a growing concern of many institutions -- model risk -- that their models are not current, accurate or effective enough.
It will take time for most banks to use cognitive systems to improve the requirements of direct-to-customer engagement, in which the requirements of personalisation and context-based interaction can still be reasonably served by current tools. But the use of cognitive systems in the front office is of high potential in terms of machine-to-human interaction, personalisation of interactions and context-based engagement.
But here is probably the most exciting part: self-service advisory and robo-advisers -- both backed by cognitive systems -- will be able to make a true business case as they improve customer recommendations.
So far, robo-advisory services remain rather basic, and still at the early stage of technology maturity. Its use is merely in rebalancing various funds to manage exposure to different asset classes, allowing for a diversification strategy and in some cases a tax-loss harvesting strategy.
They will primarily serve investors with simple diversification needs based on rebalancing and tax-loss harvesting. Over time, with growing data volumes also factoring noise from market hypes or panics, these will eventually improve accuracy and complexity. With this maturity we will see a shift from passive strategies around rebalancing towards active trading and more complex trading strategies. These active robots are likely to have a bigger impact on asset managers and return-seeking, high net worth clients with a high risk appetite rather than the mass market.
The use of cognitive capabilities with customer financial data and enterprise data will allow the financial institution to find new ways to seek revenue from unseen areas and from customer behaviour. Going forward, cognitive will even eventually help consumers deal with the overabundance of data to manage their financial lives.
Overall, we expect a steady uptake for cognitive. Similar to how highly anticipated technologies panned out, cognitive computing does not have to be a large-scale, enterprise-wide program very quickly for banks and insurers. It can be deployed in very discrete and tactical use cases. Investments, too, are not of the bet-the-bank type, since vendors fighting for mind share in this potentially hot area are willing to work closely with early adopters, including aspects of pricing, resources-sharing and reuse.
Cognitive can and should wait. Continuous learning is a key capability of machine learning. As a model is "retrained" based on new data, its behaviour and accuracy will change -- and improve. Together with other quickly emerging technologies (particularly robotic process automation and blockchain/distributed ledger technologies, which we will talk about in future instalments), cognitive technologies can be the basis on which the institution will build future architectures to handle data and analytics, process automation and decision making.
While not displacing legacy systems altogether, cognitive systems will supplant some of the functionality of legacy systems and allow institutions to greatly accelerate their transformation strategy.
Michael Araneta is the country manager of IDC Thailand.