Today, the most successful tech giants such as Google, Facebook and Amazon make business decisions informed by the wealth of data that they possess. Being "data-driven'' has become the new imperative for companies striving to become competitive in the modern landscape.
But are there downsides to this approach?
One major pitfall is machine bias. Despite popular belief, machines are prone to all kinds of biases just like humans. While certain pitfalls may lead to small errors such as inefficiencies, others can lead to more detrimental outcomes like racial profiling.
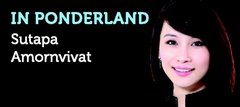
Sutapa Amornvivat, PhD, is CEO of SCB ABACUS, an AI-powered data analytics subsidiary of Siam Commercial Bank, where she previously headed the Economic Intelligence Centre and the Risk Analytics Division. She received a BA from Harvard and a PhD from MIT. email Scbabacus@scb.co.th.
Automation Intelligence (AI) systems are only as good as their training data. Thus, if built on subjective data, machines can amplify existing human bias such as racial discrimination.
Machine algorithms that are particularly prone to this bias are those used for profiling -- assessing or classifying individuals based on characteristics. Profiling algorithms like risk assessment, job placement and customer segmentation are susceptible to any kind of bias that exists within the data. This can be harmful in the context of HR analytics that employs computers to select candidates.
In a more serious case, the US justice system in some states has been using algorithms to aid its court decisions. Machines analyse how likely a defendant is to repeat bad behaviour based on data on past convictions, age, gender, race and other demographic factors. ProPublica, a non-profit news organisation, finds that the algorithm in use is biased against certain racial groups. Black defendants were twice as likely as white defendants to be misclassified as having a higher risk of reoffending.
Confirmation bias is another pitfall to beware of. Algorithms such as the Facebook news feed, which filter and display content based on user preferences, can result in a self-fulfilling prophecy where readers are only presented with information that reaffirms their views. Meanwhile, these users are methodically prevented from being challenged with opposing perspectives. In the context of politics, these algorithms can contribute the propagation of "fake news" and intensify social polarisation. The powerful influence of social media platforms can magnify the natural confirmation bias of the public masses, potentially impacting political outcomes.
Acting on data which contain biases can lead to negative outcomes. What can we do about it?
First, since algorithms are predisposed to incorporating the bias of their designers, an awareness at the creator level is necessary. Data scientists should ensure a thorough process in actively searching for hidden biases and then taking appropriate measures to remove or minimise their effects. Transparency and honesty are required when selecting the training data for machine learning algorithms. A robust evaluation scheme of a given algorithm should be in place before implementation.
Second, companies should put in place a mechanism to provide accountability. As technologies evolve and machine learning algorithms are applied to more processes, it will become increasingly difficult to hold a human accountable when something goes wrong. A well-established procedure to ensure accountability is required to provide the right incentives for the very people involved in building machines to be meticulous in cleaning up biases.
Third, establishing regulatory safeguards on use. Regulators worldwide have been tackling this issue. In December 2017, a bill was passed by New York City council to set up a dedicated task force to monitor the use of black box algorithms, and assess their impact on the public. The scope of this new auditing scheme covers algorithms used by public agencies, ranging from school placement to firefighters' building inspections.
On a larger scale, an upcoming law in Europe, the General Data Protection Regulation (GDPR), will include measures to protect consumers against these machine biases by granting people "the right to an explanation". Consumers can legally demand an explanation of the algorithms and logic behind the decisions that create material impacts on their lives. Companies including banks will need to be mindful of transparency when making machine-aided decisions.
It remains to be seen whether such regulation will prohibit the use of certain black-box AI algorithms like neural networks and deep learning. As AI technology becomes more ubiquitous, a comprehensive understanding of its inner workings has become increasingly important, and desired.
Aside from machine bias, companies should be cautious of blind spots that may accompany data-driven culture. In her talk a few years ago, Susan Athey, an economics professor at Stanford University, warned of how being data-driven could make us too short-sighted. To gain data from customers, a new product or feature sometimes undergoes A/B testing; a controlled experiment in which we observe the interaction and response of a small fraction of customers. These exploratory tests allow companies to experiment with ideas, fail fast and move on. Yet, we often forget that the results only reveal the short-term outcome. In reality, products may take time to catch on. Being too reliant on such tests can create a habit of short-termism, forgoing long-term investment.
With the right tools and technology, crucial insights can be unlocked from data. At the same time, we should be aware that the blind spots and biases within can lead us to the wrong conclusions. Real limitations to data-driven approaches exist and necessitate human oversight to ensure that they are utilised correctly and to their fullest potential.